Transforming Images into Text: A Complete Guide
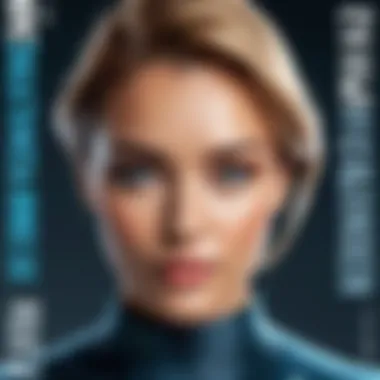
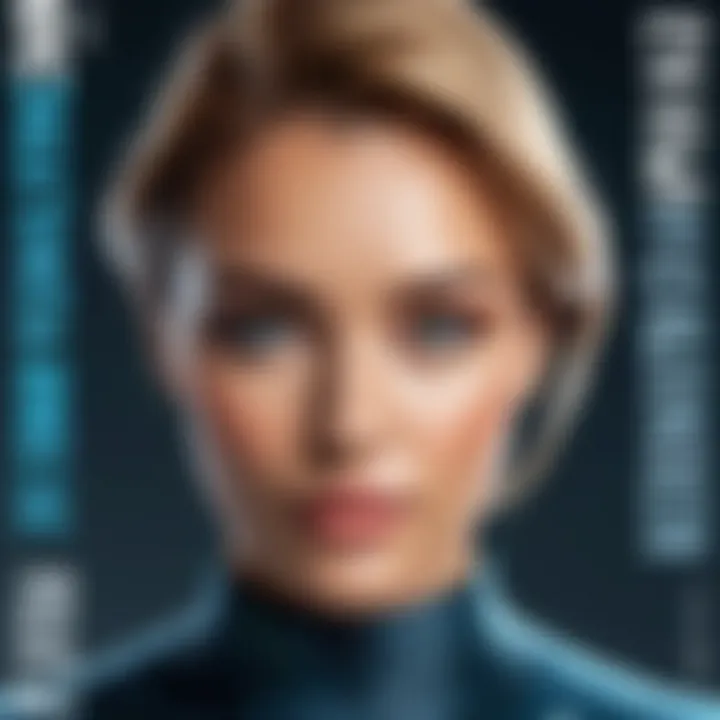
Intro
In todayâs fast-paced digital landscape, our reliance on visual data is at an all-time high. From photographs that tell stories to infographics that convey complex information, the ability to interpret and convert these visuals into text is crucial. Transforming images into textual representation isnât just about reading text; itâs about understanding context, meaning, and the nuances embedded in visual cues.
Through the integration of advanced technologies like Optical Character Recognition (OCR), machine learning, and sophisticated image processing algorithms, this unprecedented capability is now within reach of the average user. Imagine snapping a picture of a page and instantly having it transformed into editable textâa game changer for students, professionals, and anyone looking to streamline their workflow.
Consider the landscape of modern education, for instance. Students can capture notes during lectures and convert them into digital formats for better accessibility and editing. In the business realm, companies leverage these tools for data extraction from invoices and reports, enhancing productivity and accuracy. The potential applications are vast, stretching from healthcare to marketing, making the understanding of this process paramount for tech-savvy individuals.
In this guide, weâll embark on an illuminating journey, exploring the key methodologies that equip us to tackle the challenge of converting images to text. We will dissect the inner workings of various techniques, evaluate their effectiveness, and ponder the implications that arise in a world where data accessibility is increasingly essential.
Letâs delve into the first section, unraveling the intricacies of the technologies involved in this fascinating intersection of imagery and text.
Understanding the Concept
In a world where the visual reigns supreme, the translation of imagery into textual form is a task of great significance. Image to text conversion serves as a bridge between visual data and textual representation, unlocking a wealth of information hidden within images. This process becomes particularly vital in various sectors, as businesses and individuals continuously seek to enhance data accessibility and utilization. Whether itâs for digitizing old documents, improving search capabilities, or making content accessible to those with visual impairments, understanding this concept lays the groundwork for further exploration of the technologies and methodologies that drive these innovations.
What is Image to Text Conversion?
Image to text conversion is essentially the process of extracting textual information from images. This can be done through various methods and technologies, primarily focusing on Optical Character Recognition (OCR). Upon scanning an image, OCR analyzes the shapes of letters and words, converting these visuals into machine-readable text. Many tools and applications today utilize this technology, allowing users to effortlessly transform photographs of printed documents, signboards, and more into editable text. The convenience this offers canât be overstated; it opens doors for better documentation, archiving, and even aids in creating more interactive interfaces.
Historical Context and Evolution
The journey of converting images to text has evolved remarkably since its inception. Initial attempts can be traced back to the early 20th century with rudimentary technology. The landscape really changed with the introduction of OCR in the 1950s. At that time, systems like the one developed at RCA were quite simple and could only recognize limited character sets.
"OCR technology has come a long way since its nascent days, and todayâs systems are capable of interpreting various fonts, styles, and even handwriting with startling accuracy."
In the decades that followed, researchers innovated continuously, expanding OCRâs capabilities. The introduction of machine learning techniques in the late 20th century further propelled the technology, allowing algorithms to learn from substantial datasets. Today, with the integration of neural networks and advanced image processing, image to text conversion is more efficient and accurate than ever before. Presently, sectors like education, healthcare, and e-commerce benefit significantly from these advancements, illustrating the importance of understanding image to text conversion not only as a technological concept but also as a critical tool for enhancing productivity and accessibility.
Core Technologies Involved
When talking about transforming images into text, core technologies are the backbone. They dictate how effectively and accurately the conversion happens, making them pivotal in this field. Understanding these technologies not only sheds light on how the process works but also highlights their relevance in various applications, from automating data entry to enhancing accessibility for individuals with visual impairments.
Optical Character Recognition Explained
Optical Character Recognition, commonly referred to as OCR, stands as a cornerstone technology for image-to-text conversion. It serves as the bridge that connects visual information with textual formats. At its essence, OCR takes an image of text and breaks it down into recognizable letters, numbers, and symbols. This is done through a sequence of steps: image pre-processing, feature extraction, and pattern recognition.
- Image pre-processing cleans up the image, removing unwanted noise and enhancing clarity.
- Feature extraction identifies key characteristics of letters, such as lines and curves.
- Pattern recognition compares these features against a library of known characters.
The beauty of OCR lies in its growing accuracy. Modern systems can recognize not just printed text, but also cursive handwriting and a variety of languages. This adaptability makes OCR vital for tasks ranging from archiving documents to digitizing books, ensuring that important information doesn't fade away with time. It can also significantly reduce data entry errors, streamlining processes that used to be tedious and error-prone.
"Optical Character Recognition has revolutionized how we interact with digital text; itâs not just about text conversion â itâs about making information accessible."
Machine Learning and Neural Networks
Machine learning has made significant strides in enhancing the capabilities of image-to-text conversion. With the use of neural networks, algorithms can learn from vast datasets, improving their accuracy over time. Machine learning models analyze patterns and adapt based on feedback, allowing the technology to evolve continually. For instance, Convolutional Neural Networks (CNNs) are particularly effective for image recognition tasks.
These models break images down into layers, allowing for complex feature identification, such as distinguishing between different fonts or deciphering a text over a complicated background. The magic of using machine learning in this context is its ability to learn and improve; this doesn't just make it versatile, but also tailored to various requirements.
Consider the implications of this adaptability. A tool that learns from user interactions can continuously enhance its accuracy, ultimately leading to better performance over time. This stands in stark contrast to traditional OCR systems that may rely solely on static algorithms.
Image Processing Techniques
The effectiveness of converting images to text is also heavily dependent on image processing techniques. These techniques are essential for preparing the image before the OCR process kicks in. Different processing methods can dramatically affect the quality of output.
Key image processing techniques include:
- Binarization: Converting images to two colors â usually black and white â to enhance text clarity.
- Denoising: Removing undesired noise, such as blurred areas or irregular textures, to sharpen the text representation.
- Skew Correction: Adjusting the orientation of tilted images so that the text aligns properly.
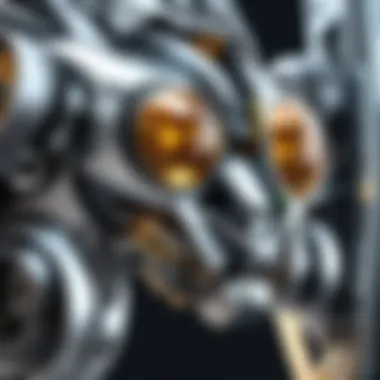
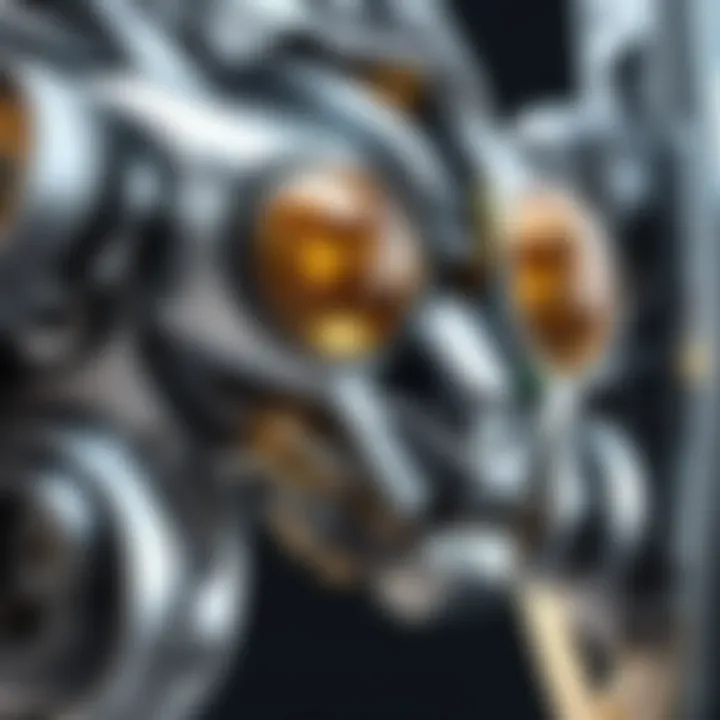
Each of these processes aims to create a clean, clear image to maximize the potential of OCR systems. Without them, even the most advanced OCR tool would struggle to deliver accurate results. As such, mastering these techniques is fundamental for anyone involved in the image-to-text conversion process, enhancing not only quality but also user experience.
In summary, the core technologies involved in transforming images to text intertwine to create a seamless transition from visual to verbal. Understanding OCR, machine learning, and image processing provides insight into the true potential and application of this transformative process.
Practical Applications
The realm of converting images into text isn't just a fascinating endeavorâit's a necessity in several industries. With the pace at which technology is advancing, practical applications of this transformation have become increasingly relevant, impacting areas like document management, accessibility, and big data analysis. At its core, the ability to turn visual content into text opens up new doors for efficiency, usability, and accessibility, making it an invaluable tool in today's digital landscape.
Use in Document Digitization
When it comes to document digitization, image-to-text conversion plays a pivotal role. Organizations, especially those with vast archives of paperwork, often rely on this technology to transition from traditional storage methods to digital databases. . Here are some factors to consider:
- Enhancing Searchability: When documents are converted to text, it becomes easy to search and retrieve information using keywords. Imagine scouring through a sea of printed pagesâtime wasted that could be better spent elsewhere.
- Space-Saving: Storing paper documents takes up valuable physical space. Digitization enables companies to switch to cloud-based solutions, freeing up office environments for more productive uses.
- Preservation: Over time, paper deteriorates, and important records can be lost forever. Digital formats maintain the information's integrity and avoid degradation.
In sum, the use of image-to-text technology in document digitization is about making information more manageable and accessible.
Applications in Accessibility Technologies
Another significant application lies in accessibility. For individuals with visual impairments, the ability to convert images into text can dramatically enhance their interaction with the world around them. By employing Optical Character Recognition and similar technologies, various tools have emerged that assist those who may otherwise struggle to read.
Consider the following tools:
- Screen Readers: Many screen readers can interpret text extracted from images, allowing visually impaired users to access content that others take for granted.
- Mobile Apps: Applications like Microsoft Seeing AI leverage image-to-text tech to provide real-time descriptions of surroundings, such as reading signage or deciphering printed material.
- Classroom Integration: Students with disabilities benefit significantly in educational contexts, where technology can help present content in more accessible forms.
Essentially, the use of image-to-text conversion in accessibility technologies is about leveling the playing field. Everyone deserves access to information, and this technology helps remove barriers.
Implications for Data Analysis
As industries continue to generate massive amounts of data, the implications for data analysis grow increasingly potent. Converting images to text can help unlock valuable insights hidden within visual data. Here are some aspects where this transformation proves beneficial:
- Sentiment Analysis: By analyzing text extracted from imagesâsuch as customer feedback formsâcompanies can gauge public sentiment about their products or services.
- Market Trends: Images containing statistical charts or consumer patterns can be converted to text, enabling analysts to more easily collate and assess market trends.
- Data Enrichment: When combined with other data points, text derived from images can add depth to datasets, improving decision-making processes.
"In a data-driven world, every byte counts. Transforming images into text helps organizations make sense of vast volumes of data, revealing insights that might otherwise stay hidden."
Challenges in Image to Text Conversion
When diving into the intricacies of converting images into text, it's vital to address the hurdles that surface throughout the process. Challenges in image to text conversion are not mere annoyances; they stem from the very nature of the images being processed and the technology employed. Understanding these challenges enhances our grasp of image processing technologies and their practical implications.
Varied Font Styles and Sizes
One of the foremost difficulties in image to text conversion lies in the diversity of font styles and sizes present in written materials. When scanning documents, fonts such as Arial, Times New Roman, or even custom typographies can lead to discrepancies in character recognition. For instance, a delicate cursive font can throw an Optical Character Recognition (OCR) engine off course, resulting in errors or omissions.
In addition to typical fonts, when fonts change size frequently within a single document, it complicates the recognition task further. Here are some notable considerations:
- Readability: Standard fonts are often designed for optimal legibility, but creative fonts might lead OCR systems to misinterpret characters.
- Mixed Fonts: A document containing multiple fonts can confuse OCR processes, leading to inconsistent results.
- Special Characters: Fonts might include characters that are non-ASCII, creating additional recognition problems.
Addressing this issue requires continuous training of OCR systems with varied datasets to boost their adaptability. Otherwise, an advanced system may still stumble on even the simplest of typographical styles.
Image Quality and Resolution Issues
The quality of the image holds significant sway over the success of text conversion efforts. Low-resolution images can hide fine details, rendering text unrecognizable.
For example, consider a document that has undergone multiple photocopies. Each copy degrades the original quality, blurring characters and causing significant challenges for conversion. Key factors to note include:
- Compression Artifacts: Images that have been heavily compressed for size may suffer from pixelationâleading to the loss of critical visual information, making accurate text extraction nearly impossible.
- Lighting Conditions: Images captured in poor lighting may also affect recognition, as shadows and glares obscure the text.
- Color Variations: Background colors opposing text colors can diminish visibility. For instance, a light grey text on a white background can be particularly challenging for OCR systems.
It often becomes a game of cat and mouseâusers must often clean up images manually before they can even begin to think about accurate conversion.
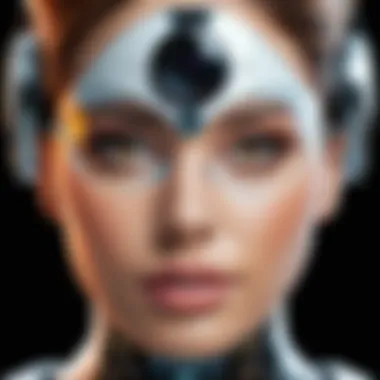
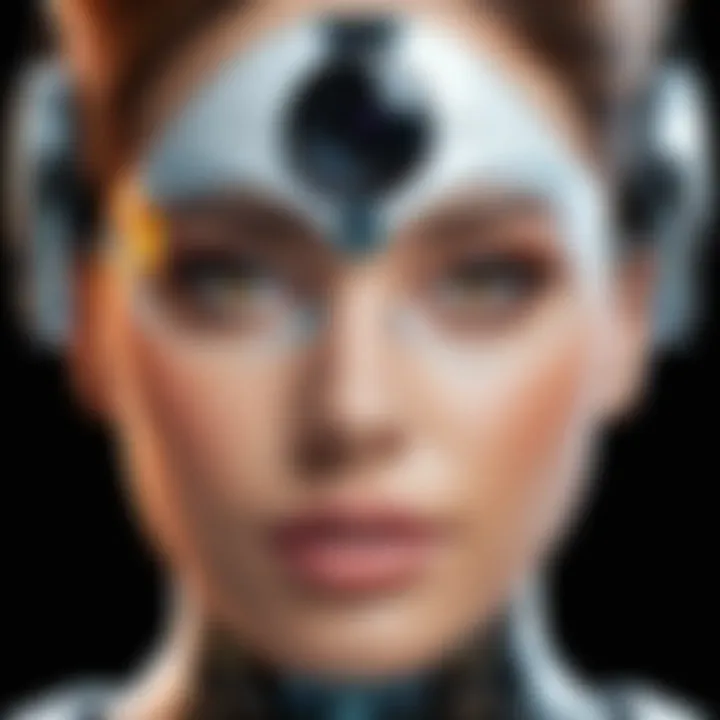
Complex Layouts and Graphics
Another significant hurdle arises when handling documents with intricate layouts or graphical elements. Many documents are not just plain text; they feature images, tables, and figures that can disrupt an OCR tool's capacity to correctly parse text.
Take a typical magazine page: scattered text sections alongside images, infographics, or sidebars can lead OCR tools to misplace or misinterpret text. Critical considerations in this area include:
- Text Flow: In multi-column layouts, determining the direction of text flow is often challenging for unequipped OCR systems.
- Overlapping Elements: Text that overlaps with images or graphic patterns can become jumbled, making it harder to distill clear content.
- Mixed Media: The presence of different media types (like scanned handwritten notes) exacerbates these issues, leading to an even trickier extraction process.
Ultimately, to improve the success of image to text conversion, developers need to fine-tune their algorithms to recognize and contextually understand these diverse formats better.
"With each hurdle comes the opportunity to innovate and refine our processes"
Evaluating Effectiveness
In the realm of image-to-text conversion, evaluating effectiveness is not just a sideline conversation; it stands as the backbone of any successful implementation. This allows users to discern which tools or technologies manage to hit the nail on the head and which ones fall short. Effectiveness embodies the quality and accuracy of the text output from images, which directly influences usabilityâan aspect that should never be overlooked. Understanding how various solutions ensure high levels of precision is crucial for anyone looking to bring efficiency into their workflows.
Moreover, effectiveness evaluation helps in identifying the most appropriate tools for specific needs. Itâs not merely about getting a readable output; one must consider factors like speed, adaptability, and even capacity to withstand various image conditions, such as clarity, orientation, and complexity. This broad view is essential for tech aficionados and daily users alike since it navigates them through a sea of options and potential pitfalls.
Performance Metrics
Performance metrics act as the yardsticks by which the quality of image to text conversion tools can be judged. These are significant because they reveal how well a tool performs relative to others. Metrics such as accuracy, speed, and efficiency paint a fuller picture. Accuracy measures the reliability of the transcribed text against the original image, while speed deals with the time taken to convert.
Here are some common performance metrics to consider:
- Accuracy Rate: Represents the percentage of correctly identified characters or words. The closer the measurement is to 100%, the better the tool.
- Processing Time: Calculates how long it takes from image input to text output. Faster is typically better, especially for large batches.
- Error Rate: This tracks how often mistakes happen during conversions, providing insight into the reliability of the tool.
- Resource Usage: This metric can inform how efficiently a tool runs, taking into account CPU and memory consumption.
Evaluating these metrics allows one to make a more informed decision about which technology best suits specific requirements.
Comparison of Available Tools
The market offers a vast array of tools for converting images to text, and sorting through these options can feel like searching for a needle in a haystack. However, a thoughtful comparisons reveals the strength and weaknesses of various platforms. From robust, enterprise-level systems to simple, user-friendly apps, each has its place in the ecosystem.
Key Tools to Consider
- Adobe Acrobat DC: A commercial powerhouse that not only performs OCR but also offers document editing capabilities. Its integration in workflows adds value for users who often deal with PDFs.
- ABBYY FineReader: Known for high accuracy and a range of supported languages, making it a popular pick in corporate settings.
- Tesseract OCR: An open-source option highly favored by tech enthusiasts and developers, Tesseract provides flexibility for custom solutions.
- Google Drive: A hidden gem, Google Driveâs OCR functionality is easy to use and integrated into the document management interface, providing instant accessibility.
Considerations When Choosing
- User Experience: Is the interface intuitive or does it require a steep learning curve?
- Cost: Are the financial commitments aligned with the functions youâre getting? Open-source does not always mean inferior, and proprietaries do not always guarantee excellence.
- Updates and Support: Does the tool receive frequent updates and community support? This feature can be advantageous in staying current with emerging technologies.
In short, evaluating effectiveness is about more than just picking a tool; itâs about understanding what metrics matter for your needs and how various tools stack up against those metrics. It allows one to tailor the image-to-text conversion process to personal or organizational requirements, enhancing productivity down the line.
"In today's fast-paced environment, choosing the right image conversion tool goes beyond mere functionality; it is a step toward achieving greater efficiency and precision in digital workflows."
Choosing the Right Tool
Choosing the appropriate tool for converting images into text is critical in ensuring that the process is efficient and yields accurate results. With a myriad of options available on the market, understanding the different factors at play can help users make an informed decisionâwhether for business, education, or daily life. The right tool not only enhances productivity but also improves the quality of the output. Various elements come into play when selecting a software solution, including the nature of the images, the end purpose of the text output, and the user's technical proficiency.
When weighing options, consider the following benefits and considerations:
- Quality of Output: Not all tools are made equal. Some may excel with standard fonts while struggling with complex layouts or distorted images. Look for solutions that provide clear image outputs and high accuracy rates.
- User Experience: A tool that is easy to navigate saves time and frustration. More intuitive designs can lead to quicker learning curves, allowing users to get started without heavy training.
- Integration Capabilities: The ability to seamlessly connect with other software can streamline workflows. If your operations rely on certain platforms, check if the tool of interest has built-in integrations.
- Customization Options: Depending on your specific needs, some applications might allow modifications to settings, which can optimize the conversion process according to unique requirements.
"A good tool adapts to your needs, and not the other way around."
By taking these factors into account, users can select tools that aid not only in efficiency but also in achieving higher accuracy levels in image to text conversion.
Open Source vs. Proprietary Software
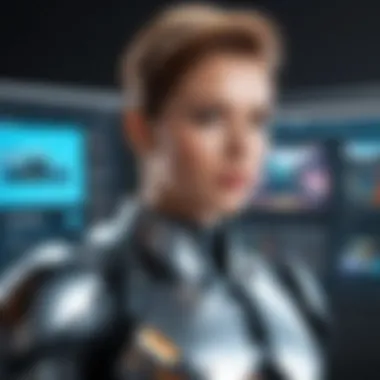
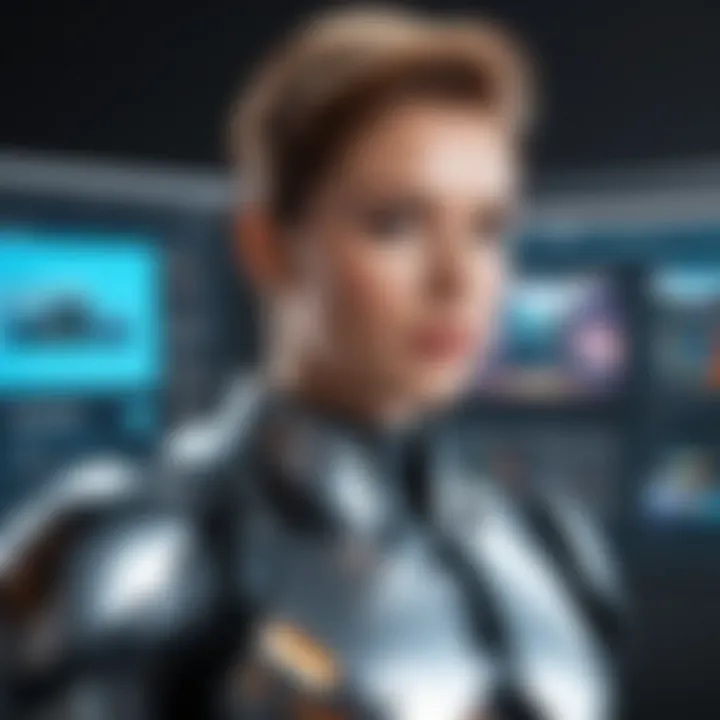
Navigating the landscape between open source and proprietary software can be daunting, especially for those new to technology. Open source software is freely available for anyone to use, modify, and distribute. This encourages community-driven developments and often results in rapid improvements made by passionate developers. Examples include Tesseract and OCRmyPDF.
On the flip side, proprietary software is typically developed by specific companies who charge for their products. These tools, like Adobe Acrobat or Omnipage, often come with customer support, regular updates, and more polished designs suited for professional environments. Here are some key considerations when choosing between the two options:
- Cost: Open-source solutions are free but may require more setup time. Proprietary tools usually come with a cost, which may include hidden fees for advanced features.
- Community Support: Open-source tools rely heavily on community support, which can sometimes be less reliable. In contrast, proprietary options often boast robust customer service.
- Updates and Features: Proprietary software frequently receives updates for bugs and new features. Open-source tools may also offer updates, though the timeline can be less predictable.
In choosing between the two, it is essential to analyze your needs and the resources available. Depending on the task at hand, one may serve better than the other, or even a combination of both may be necessary.
User-Friendly Interfaces for Beginners
For beginners, diving into the world of image to text conversion can often feel overwhelming. The complexity of certain tools can lead to frustration and even abandonment of the task. Thus, selecting an application with a user-friendly interface becomes paramount. These interfaces offer a smoother entry into technology without extensive training.
Hereâs what to look for in user-friendly tools:
- Simple Navigation: The layout should be intuitive. Menus should be clearly labeled and functions easily accessible without a maze of options.
- Guided Steps: Tools that offer step-by-step instructions or tutorials can significantly aid newcomers. It reduces the learning curve and boosts users' confidence.
- Visual Feedback: An interface that provides immediate visual feedback after an action can help users understand processes better and feel more connected to the tool.
- Help Resources: Availability of FAQs or support forums enhances user experience, allowing beginners to seek help when needed. Extensive documentation or community discussions can bridge knowledge gaps.
By choosing software with such attributes, beginners can transition smoothly into utilizing image to text conversion tools without hitting too many bumps along the way.
Future of Image to Text Conversion
The prospects for transforming imagery into text are not just an incremental step in tech evolution; they represent a shift in how we interact with data and information. With the advancements in technology, the ability to seamlessly convert visuals into readable text isn't merely beneficialâitâs becoming increasingly indispensable in various sectors. This shift offers significant advantages, from improved efficiency in data entry to enhanced accessibility for users facing challenges with traditional content formats.
As more businesses and individuals recognize the value of managing large volumes of visual data, the future holds the promise of tools that enable quicker and more accurate conversions. Beyond everyday applications, this technology plays a critical role in augmenting digital inclusivity and usability for audiences that may otherwise be restricted by visual obstacles. The evolution of this process speaks volumes about our collective ability to harness technology to bridge gaps between different forms of media.
Advancements in AI and OCR
Artificial Intelligence (AI) is spearheading remarkable changes in the realm of Optical Character Recognition (OCR). Once limited to simple text extraction, current AI-driven OCR systems employ machine learning techniques to enhance their accuracy and adaptability. They can now recognize handwritten notes, varied font styles, and even stylized graphics with surprising competence.
Recent advancements such as convolutional neural networks (CNNs) are reshaping how OCR processes images. These networks analyze images like a human eye would, discerning patterns and structures with a nuanced understanding that earlier technologies lacked. Consequently, they improve not just the correctness of extraction but also the contextual awareness regarding different symbols and arrangements in an image. The broader implications of these advancements are extensive, suggesting a future where automatically generated text may retain inherent context and meaning, thus making a significant leap from simple conversions.
But that's not all! Integration of deep learning techniques in OCR is allowing software to learn from past errors and continuously improve over time. This could lead us towards systems that intuitively adjust to user preferences, becoming more personalized and effective with each use.
Potential for Integration with Other Technologies
Looking ahead, the harmony between image-to-text conversion and other technologies appears promising. The integration of OCR with Natural Language Processing (NLP) is a compelling example. By enabling OCR systems to not only extract text but also comprehend it, we start to see phenomenal capabilities emerge. For instance, users could upload an image containing a legal document, and the system could not only convert it to text but analyze it to summarize essential points.
Furthermore, the fusion with augmented reality (AR) holds the potential to redefine user engagement. Picture someone wearing AR glasses that can interpret street signs in real time, translating them into different languages as needed. The ability to change visual inputs into comprehensible data on-the-fly opens doors to numerous applications in travel, education, and more.
"The future of this field is not just in making images legible but elevating our entire interaction with information across mediums."
Through these efforts, we're not just improving existing processes but expanding horizons for ingenuity and accessibility, reminding us that images hold more than just beautyâthey carry stories waiting to be told.
End
The conclusion of this article serves as a vital anchor point, summarizing the essential components of transforming images to text while reflecting on the journey we've undertaken through various methods and technologies. The importance of understanding the intricacies of this topic cannot be overstated, especially in a world that is increasingly reliant on data accessibility and usability. The ability to effectively convert images into text is not merely an academic exercise; it's a necessary skill in todayâs digital landscape, enabling more efficient data processing and retrieval.
In this guide, we underscored several specific elements that are pivotal in making image-to-text conversion efficient and effective. The first is the advancement in Optical Character Recognition (OCR) technology, which has made significant leaps, allowing for better recognition of complex fonts and handwritten characters alike. This development has opened doors in diverse sectors from education to business, allowing for smooth digitization of physical records.
Secondly, we discussed the impacts of machine learning, noting how neural networks are trained to adapt to new types of text and layouts, improving accuracy and speed in conversion tasks. This adaptability is invaluable as it helps in processing a broader range of images and contexts, which is crucial in achieving correct outputs.
Key Takeaways
- The transformation of image to text is essential for enhancing data accessibility, making information more retrievable and usable.
- Advanced OCR technologies enable better recognition of varied fonts and formats, contributing to higher accuracy.
- Machine learning plays a significant role by constantly improving the algorithms that make image-to-text conversion effective.
- Users should evaluate their needs carefully, considering both practical applications and the technology's limitations when selecting tools for conversion.
The Path Forward
The path ahead in image-to-text conversion is promising. Itâs clear that as technology advances, we can anticipate even greater integration of AI and OCR, providing smarter and more adaptable solutions. Innovations signify a movement towards seamless usability where converting images to text could become instantaneous, potentially through real-time applications in mobile settings.
Moreover, merging image-to-text technology with other platformsâsuch as augmented reality or augmented data analysis toolsâcould create a convergence that benefits industries far and wide. As tech-savvy individuals and early adopters, it's important to stay abreast of these developments. By recognizing the potential challenges, such as varied image quality and layout complexities, we can prepare to embrace the evolution that lies ahead effectively.
"The future of image to text conversion will not just be about technology. It will be about how we use these advancements to make information accessible for all."
This forward-thinking approach will guide us to leverage the emerging technologies effectively, ensuring that our endeavours in transforming imagery into text are optimized for the next generation of users.